StableVITON: Learning Semantic Correspondence with Latent Diffusion Model for Virtual Try-On
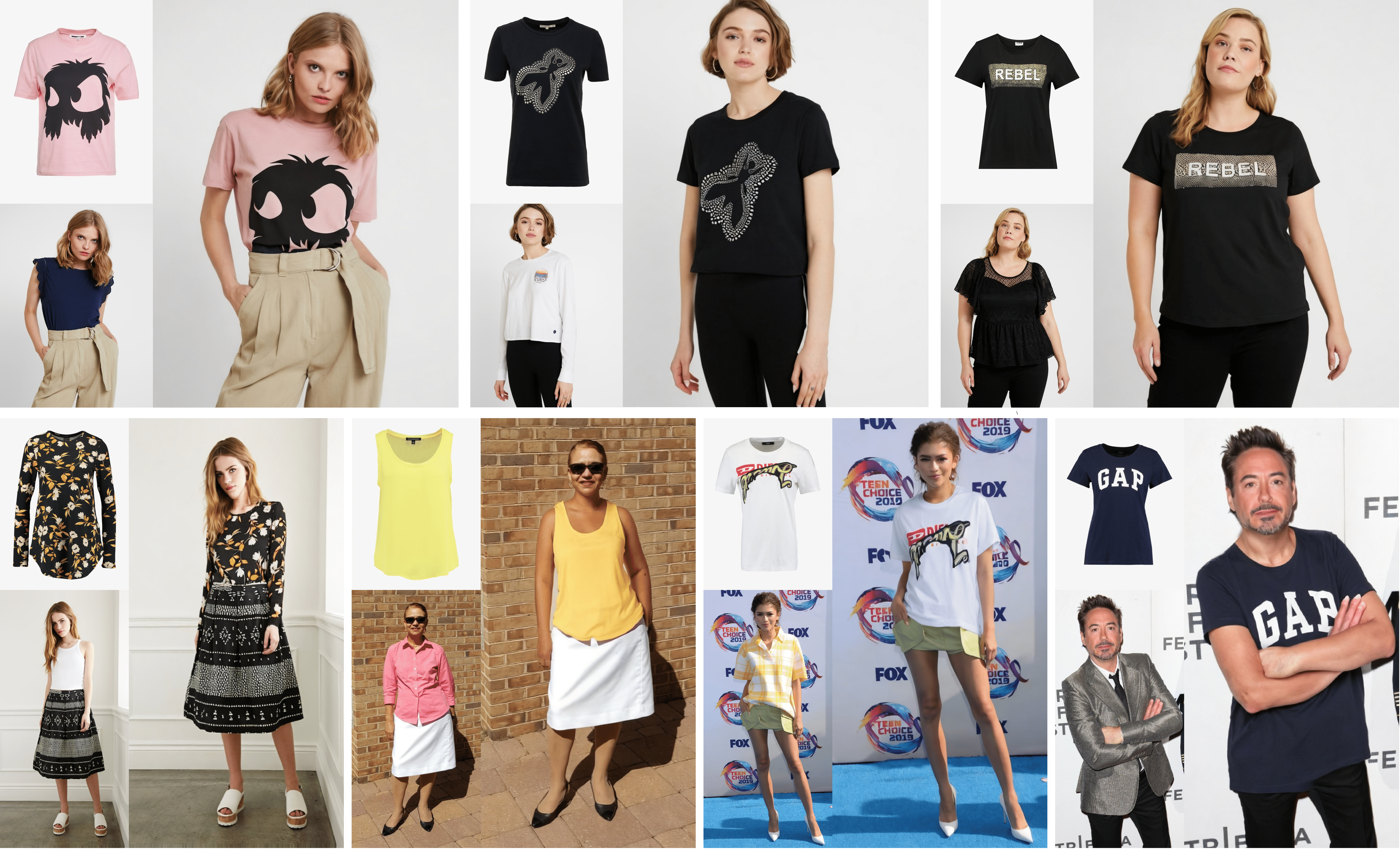
Given a clothing image and a person image, an image-based virtual try-on aims to generate a customized image that appears natural and accurately reflects the characteristics of the clothing image. In this work, we aim to expand the applicability of the pre-trained diffusion model so that it can be utilized independently for the virtual try-on task. The main challenge is to preserve the clothing details while effectively utilizing the robust generative capability of the pre-trained model. In order to tackle these issues, we propose StableVITON, learning the semantic correspondence between the clothing and the human body within the latent space of the pre-trained diffusion model in an end-to-end manner. Our proposed zero cross-attention blocks not only preserve the clothing details by learning the semantic correspondence but also generate high-fidelity images by utilizing the inherent knowledge of the pre-trained model in the warping process. Through our proposed novel attention total variation loss and applying augmentation, we achieve the sharp attention map, resulting in a more precise representation of clothing details. StableVITON outperforms the baselines in qualitative and quantitative evaluation, showing promising quality in arbitrary person images..
For the virtual try-on task, StableVITON additionally takes three conditions: agnostic map, agnostic mask, and dense pose, as the input of the pre-trained U-Net, which serves as the query (Q) for the cross-attention. The feature map of the clothing is used as the key (K) and value (V) for the cross-attention and is conditioned on the UNet, as depicted in (b).
The attention mechanism in the latent space performs patch-wise warping by activating each token corresponding to clothing alignment within the generation region. Moreover, to further sharpen attention maps, we propose a novel attention total variation loss and apply the augmentation, which yields improved preservation of clothing details. By not impairing the pre-trained diffusion model, this architecture generates high-quality images even when images with complex backgrounds are provided, only using an existing virtual try-on dataset.
Generated results for VITON-HD, DressCode, SHHQ-1.0, and web-crawled images. All generated outputs were produced using StableVITON, which was trained on the VITON-HD training dataset.
@article{kim2023stableviton,
title={StableVITON: Learning Semantic Correspondence with Latent Diffusion Model for Virtual Try-On},
author={Kim, Jeongho and Gu, Gyojung and Park, Minho and Park, Sunghyun and Choo, Jaegul},
journal={arXiv preprint arXiv:2312.01725},
year={2023}
}
Project page template is borrowed from DreamBooth.
Acknowledgements. Sunghyun Park is the corresponding author.